We show that the empirical Christoffel function associated with a cloud of finitely many points sampled from a distribution, can provide a simple tool for supervised classification in data analysis, with good generalization properties.
Nous montrons que la fonction de Christoffel empirique associée à un échantillon fini de points peut fournir un outil simple pour la classification supervisée en analyse de données, avec de bonnes propriétés de généralisation.
Révisé le :
Accepté le :
Publié le :
Jean B. Lasserre 1
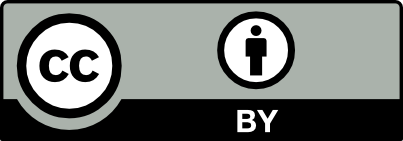
@article{CRMATH_2022__360_G8_919_0, author = {Jean B. Lasserre}, title = {On the {Christoffel} function and classification in data analysis}, journal = {Comptes Rendus. Math\'ematique}, pages = {919--928}, publisher = {Acad\'emie des sciences, Paris}, volume = {360}, year = {2022}, doi = {10.5802/crmath.358}, language = {en}, }
Jean B. Lasserre. On the Christoffel function and classification in data analysis. Comptes Rendus. Mathématique, Volume 360 (2022), pp. 919-928. doi : 10.5802/crmath.358. https://comptes-rendus.academie-sciences.fr/mathematique/articles/10.5802/crmath.358/
[1] Data-Driven Science and Engineering: Machine Learning, Dynamical Systems, and Control, Cambridge University Press, 2019
[2] Sorting out typicality via the inverse moment matrix SOS polynomial, Advances in Neural Information Processing Systems (2016), pp. 190-198
[3] The empirical Christoffel function with applications in data analysis, Adv. Comput. Math., Volume 45 (2019) no. 3, pp. 1439-1468
[4] The Christoffel–Darboux Kernel for Data Analysis, Cambridge Monographs on Applied and Computational Mathematics, 38, Cambridge University Press, 2022 | Zbl
[5] Semi-algebraic approximation using Christoffel–Darboux kernel, Constr. Approx., Volume 54 (2021) no. 3, pp. 391-429
Cité par Sources :
Commentaires - Politique