This paper revisits the different arts of engineering. The art of modeling for describing the behavior of complex systems from the solution of partial differential equations that are expected to govern their responses. Then, the art of simulation concerns the ability of solving these complex mathematical objects expected to describe the physical reality as accurately as possible (accuracy with respect to the exact solution of the models) and as fast as possible. Finally, the art of decision making needs to ensure accurate and fast predictions for efficient diagnosis and prognosis. For that purpose physics-informed digital twins (also known as Hybrid Twins) will be employed, allying real-time physics (where complex models are solved by using advanced model order reduction techniques) and physics-informed data-driven models for filling the gap between the reality and the physics-based model predictions. The use of physics-aware data-driven models in tandem with physics-based reduced order models allows us to predict very fast without compromising accuracy. This is compulsory for diagnosis and prognosis purposes.
Accepté le :
Première publication :
Publié le :
Francisco Chinesta 1, 2 ; Elias Cueto 3
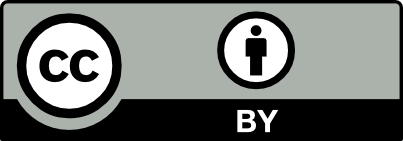
@article{CRMECA_2023__351_S3_121_0, author = {Francisco Chinesta and Elias Cueto}, title = {Conciliating accuracy and efficiency to empower engineering based on performance: a short journey}, journal = {Comptes Rendus. M\'ecanique}, pages = {121--133}, publisher = {Acad\'emie des sciences, Paris}, volume = {351}, number = {S3}, year = {2023}, doi = {10.5802/crmeca.188}, language = {en}, }
TY - JOUR AU - Francisco Chinesta AU - Elias Cueto TI - Conciliating accuracy and efficiency to empower engineering based on performance: a short journey JO - Comptes Rendus. Mécanique PY - 2023 SP - 121 EP - 133 VL - 351 IS - S3 PB - Académie des sciences, Paris DO - 10.5802/crmeca.188 LA - en ID - CRMECA_2023__351_S3_121_0 ER -
%0 Journal Article %A Francisco Chinesta %A Elias Cueto %T Conciliating accuracy and efficiency to empower engineering based on performance: a short journey %J Comptes Rendus. Mécanique %D 2023 %P 121-133 %V 351 %N S3 %I Académie des sciences, Paris %R 10.5802/crmeca.188 %G en %F CRMECA_2023__351_S3_121_0
Francisco Chinesta; Elias Cueto. Conciliating accuracy and efficiency to empower engineering based on performance: a short journey. Comptes Rendus. Mécanique, Volume 351 (2023) no. S3, pp. 121-133. doi : 10.5802/crmeca.188. https://comptes-rendus.academie-sciences.fr/mecanique/articles/10.5802/crmeca.188/
[1] The History of Crash Simulation, 2019 (https://www.youtube.com/watch?v=NU3cB9ArSZg)
[2] Nonlinear dimensionality reduction, Information Science and Statistics, Springer, 2007 | DOI
[3] Nonlinear dimensionality reduction by Locally Linear Embedding, Science, Volume 290 (2000) no. 5500, pp. 2323-2326 | DOI
[4] Visualizing data using t-SNE, J. Mach. Learn. Res., Volume 9 (2008), pp. 2579-2605 | Zbl
[5] Model Order Reduction, Encyclopedia of Computational Mechanics Second Edition (Erwin Stein; Rene de Borst; Tom Hughes, eds.), John Wiley & Sons, 2017, pp. 1-36 | DOI
[6] Certified real-time solution of the parametrized steady incompressible Navier–Stokes equations: Rigorous reduced-basis a posteriori error bounds, Int. J. Numer. Methods Fluids, Volume 47 (2005) no. 8-9, pp. 773-788 | DOI | MR | Zbl
[7] A Posteriori error bounds for reduced-basis approximation of parametrized noncoercive and nonlinear elliptic partial differential equations, Proceedings of the 16th AIAA Computational Fluid Dynamics Conference, American Institute of Aeronautics and Astronautics, Inc. (2003) | DOI
[8] A Priori convergence theory for reduced-basis approximations of single-parameter elliptic partial differential equations, J. Sci. Comput., Volume 17 (2002) no. 1-4, pp. 437-446 | DOI | MR | Zbl
[9] Global a priori convergence theory for reduced-basis approximation of single-parameter symmetric coercive elliptic partial differential equations, C. R. Math. Acad. Sci. Paris, Volume 335 (2002) no. 3, pp. 289-294 | DOI | MR
[10] A priori hyperreduction method: an adaptive approach, J. Comput. Phys., Volume 202 (2005) no. 1, pp. 346-366 | DOI | Zbl
[11] Accelerated mesh sampling for the hyper reduction of nonlinear computational models, Int. J. Num. Meth. Engrg., Volume 109 (2017) no. 12, pp. 1623-1654 | DOI | MR
[12] An “empirical interpolation” method: Application to efficient reduced-basis discretization of partial differential equations, C. R. Math. Acad. Sci. Paris, Volume 339 (2004) no. 9, pp. 667-672 | DOI | Numdam | MR | Zbl
[13] Nonlinear model order reduction via discrete empirical interpolation, SIAM J. Sci. Comput., Volume 32 (2010) no. 5, pp. 2737-2764 | DOI | Zbl
[14] Tensor representation of on-linear models using cross approximations, J. Sci. Comput., Volume 81 (2019), pp. 22-47 | DOI | MR | Zbl
[15] Incremental dynamic mode decomposition: A reduced-model learner operating at the low-data limit, C. R. Méc. Acad. Sci. Paris, Volume 347 (2019) no. 11, pp. 780-792 | DOI
[16] Dynamic mode decomposition of numerical and experimental data, J. Fluid Mech., Volume 656 (2010), pp. 5-28 | DOI | MR | Zbl
[17] A data-driven approximation of the Koopman operator: extending dynamic mode decomposition, J. Nonlinear Sci., Volume 25 (2015) no. 6, pp. 1307-1346 | DOI | MR | Zbl
[18] Learning data-driven reduced elastic and inelastic models of spot-welded patches, Mechanics & Industry,, Volume 22 (2021), 32, 17 pages | DOI
[19] Learning stable reduced-order models for hybrid twins. Data Centric Engineering, Data-Centric Engineering, Volume 2 (2021), E10 | DOI
[20] Modeling and control of physical processes using Proper Orthogonal Decomposition, Mathematical and Computer Modelling, Volume 33 (2001) no. 1-3, pp. 223-236 | DOI | Zbl
[21] Interpolation method for adapting reduced-order models and application to aeroelasticity, AIAA J., Volume 46 (2008) no. 7, pp. 1803-1813 | DOI
[22] Nonlinear Computational Structural Mechanics. New Approaches and Non-Incremental Methods of Calculation, Mechanical Engineering Series, Springer, 1999 | DOI
[23] PGD-Based Computational Vademecum for Efficient Design, Optimization and Control, Arch. Comput. Methods Eng., Volume 20 (2013) no. 1, pp. 31-59 | DOI | MR | Zbl
[24] The Proper Generalized Decomposition for Advanced Numerical Simulations. A primer, SpringerBriefs in Applied Sciences and Technology, Springer, 2014 | DOI
[25] Discovering governing equations from data by sparse identification of nonlinear dynamical systems, Proc. Natl. Acad. Sci. USA, Volume 113 (2016) no. 15, pp. 3932-3937 | DOI | MR | Zbl
[26] Regression shrinkage and selection via the lasso, J. R. Stat. Soc., Ser. B, Stat. Methodol., Volume 58 (1996) no. 1, pp. 267-288 | DOI | MR | Zbl
[27] Non-intrusive Sparse Subspace Learning for parametrized problems, Arch. Comput. Methods Eng., Volume 26 (2019) no. 2, pp. 303-326 | DOI | MR
[28] A multi-dimensional data-driven sparse identification technique: the sparse Proper Generalized Decomposition, Complexity, Volume 2018 (2018), 5608286 | DOI | Zbl
[29] PGD-based advanced nonlinear multiparametric regressions for constructing metamodels at the scarce-data limit, Adv. Model. and Simul. in Eng. Sci., Volume 10 (2023) no. 4 | DOI
[30] Support Vector Regression, Efficient Learning Machines: Theories, Concepts, and Applications for Engineers and System Designers, Apress, 2015, pp. 67-80 | DOI
[31] Decision Tree primer, 2002 (https://www.public.asu.edu/~kirkwood/DAStuff/refs/decisiontrees/index.html)
[32] Random Forests, Mach. Learn., Volume 45 (2001), pp. 5-32 | DOI | Zbl
[33] Deep learning in neural networks: An overview, Neural Netw., Volume 61 (2015), pp. 85-117 | DOI
[34] Deep learning, Adaptive Computation and Machine Learning, MIT Press, 2016
[35] Physics informed deep learning (part I): data-driven solutions of nonlinear partial differential equations (2017) (https://arxiv.org/abs/1711.10561)
[36] Physics informed deep learning (part II): data-driven discovery of nonlinear partial differential equations (2017) (https://arxiv.org/abs/1711.10566)
[37] Thermodynamically consistent data-driven computational mechanics, Comput. Mech., Volume 31 (2019), pp. 239-253 | DOI | MR
[38] Learning corrections for hyper-elastic models from data, Front. Mater., Volume 6 (2019) | DOI
[39] Deep learning of thermodynamics-aware reduced-order models from data, Comput. Methods Appl. Mech. Eng., Volume 379 (2021), 113763 | DOI | MR | Zbl
[40] Learning non-Markovian physics from data, Journal of Computational Physics, Volume 428 (2021), 109982 | DOI | MR
[41] Learning slosh dynamics by means of data, Comput. Mech., Volume 64 (2019), pp. 511-523 | DOI | MR | Zbl
[42] Digital twins that learn and correct themselves, Int. J. Numer. Methods Eng., Volume 123 (2020) no. 13, pp. 3034-3044 | DOI
[43] Dynamics and thermodynamics of complex fluids. I. Development of a general formalism, Phys. Rev. E, Volume 56 (1997) no. 6, pp. 6620-6632 | DOI | MR
[44] Numerical experiments on unsupervised manifold learning applied to mechanical modeling of materials and structures, Comptes Rendus. Mécanique, Volume 348 (2020) no. 10-11, pp. 937-958 | DOI
[45] Autoencoders, minimum description length and Helmholtz free energy, Advances in Neural Information Processing Systems, Volume 6, Morgan-Kaufmann, 1993, pp. 3-10
[46] Virtual, Digital and Hybrid Twins: A New Paradigm in Data-Based Engineering and Engineered Data, Arch. Comput. Methods Eng., Volume 27 (2020), pp. 105-134 | DOI | MR
[47] An Augmented Reality platform for interactive aerodynamic design and analysis, Int. J. Numer. Methods Eng., Volume 138 (2019), pp. 125-138 | DOI | MR
Cité par Sources :
Commentaires - Politique