A self-learning approach for optimal feedback gains for finite-horizon nonlinear continuous time control systems is proposed and analysed. It relies on parameter dependent approximations to the optimal value function obtained from a family of universal approximators. The cost functional for the training of an approximate optimal feedback law incorporates two main features. First, it contains the average over the objective functional values of the parametrized feedback control for an ensemble of initial values. Second, it is adapted to exploit the relationship between the maximum principle and dynamic programming. Based on universal approximation properties, existence, convergence and first order optimality conditions for optimal neural network feedback controllers are proved.
Révisé le :
Accepté le :
Première publication :
Publié le :
Mots clés : optimal feedback control, neural networks, Hamilton–Jacobi–Bellman equation, self-learning, reinforcement learning
Karl Kunisch 1, 2 ; Daniel Walter 3
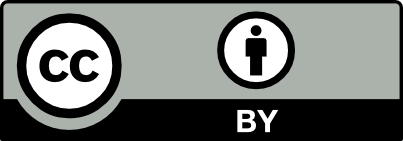
@article{CRMECA_2023__351_S1_535_0, author = {Karl Kunisch and Daniel Walter}, title = {Optimal feedback control of dynamical systems via value-function approximation}, journal = {Comptes Rendus. M\'ecanique}, pages = {535--571}, publisher = {Acad\'emie des sciences, Paris}, volume = {351}, number = {S1}, year = {2023}, doi = {10.5802/crmeca.199}, language = {en}, }
TY - JOUR AU - Karl Kunisch AU - Daniel Walter TI - Optimal feedback control of dynamical systems via value-function approximation JO - Comptes Rendus. Mécanique PY - 2023 SP - 535 EP - 571 VL - 351 IS - S1 PB - Académie des sciences, Paris DO - 10.5802/crmeca.199 LA - en ID - CRMECA_2023__351_S1_535_0 ER -
Karl Kunisch; Daniel Walter. Optimal feedback control of dynamical systems via value-function approximation. Comptes Rendus. Mécanique, Volume 351 (2023) no. S1, pp. 535-571. doi : 10.5802/crmeca.199. https://comptes-rendus.academie-sciences.fr/mecanique/articles/10.5802/crmeca.199/
[1] Controlled Markov processes and viscosity solutions, Stochastic Modelling and Applied Probability, 25, Springer, 2006 | Zbl
[2] Tensor decomposition methods for high-dimensional Hamilton-Jacobi-Bellman equations, SIAM J. Sci. Comput., Volume 43 (2021) no. 3, p. A1625-A1650 | DOI | MR | Zbl
[3] Semiglobal optimal feedback stabilization of autonomous systems via deep neural network approximation, ESAIM, Control Optim. Calc. Var., Volume 27 (2021), 16 | MR | Zbl
[4] A neural network approach applied to multi-agent optimal control (2021) (preprint, arXiv:2104.03270) | DOI
[5] An adaptive sparse grid semi-lagrangian scheme for first order Hamilton-Jacobi Bellman equations, J. Sci. Comput., Volume 55 (2013) no. 3, pp. 575-605 | DOI | MR | Zbl
[6] Numerical methods for Hamilton-Jacobi type equations (Handbook of Numerical Analysis), Volume 17, North-Holland, 2016, pp. 603-626 | DOI | MR
[7] Hamilton-Jacobi-Bellman equations: Numerical Methods and Applications in Optimal Control, Radon Series on Computational and Applied Mathematics, 21, Walter de Gruyter, 2018 | DOI
[8] Polynomial approximation of high-dimensional Hamilton-Jacobi-Bellman equations and applications to feedback control of semilinear parabolic PDEs, SIAM J. Sci. Comput., Volume 40 (2018) no. 2, p. a629-a652 | DOI | MR | Zbl
[9] Approximative policy iteration for exit time feedback control problems driven by stochastic differential equations using tensor train format (2020) (preprint, arXiv:2010.04465) | DOI
[10] Hopf formula and multitime Hamilton-Jacobi equations, Proc. Am. Math. Soc., Volume 96 (1986), pp. 79-84 | DOI | MR | Zbl
[11] Algorithm for Hamilton-Jacobi equations in density space via a generalized Hopf formula (2018) (preprint, arXiv:1805.01636) | DOI
[12] Optimal feedback law recovery by gradient-augmented sparse polynomial regression, J. Mach. Learn. Res., Volume 22 (2021), 48 | MR | Zbl
[13] Adaptive deep learning for high-dimensional Hamilton-Jacobi-Bellman equations, SIAM J. Sci. Comput., Volume 43 (2021) no. 2, p. a1221-a1247 | DOI | MR | Zbl
[14] Reinforcement Learning and Optimal Control, Athena Scientific optimization and computation series, 1, Athena Scientific, 2019
[15] Reinforcement learning and adaptive dynamic programming for feedback control, IEEE Circuits Syst. Mag., Volume 9 (2009) no. 3, pp. 32-50 | DOI
[16] A tour of reinforcement learning: The view from continuous control (2018) (preprint, arXiv:1806.09460) | DOI
[17] Neural networks in feedback control systems, Mechanical Engineers’ Handbook, Volume 3, Wiley, 2014, pp. 843-894
[18] Semi-Lagrangian approximation schemes for linear and Hamilton-Jacobi equations, Other Titles in Applied Mathematics, 133, Society for Industrial and Applied Mathematics, 2014 | Zbl
[19] Approximation theory of the MLP model in neural networks, Acta Numerica, Volume 8 (1999), pp. 143-195 | DOI | MR | Zbl
[20] Approximation capabilities of multilayer feedforward networks, Neural Networks, Volume 4 (1991) no. 2, pp. 251-257 | DOI
[21] Vector measures, Mathematical Surveys, 15, American Mathematical Society, 1977 | DOI | Zbl
[22] Mapping theorems for Sobolev spaces of vector-valued functions, Stud. Math., Volume 240 (2018) no. 3, pp. 275-299 | DOI | MR | Zbl
[23] Two-point step size gradient methods, IMA J. Numer. Anal., Volume 8 (1988) no. 1, pp. 141-148 | DOI | MR | Zbl
Cité par Sources :
Commentaires - Politique