[Quelques observations sur le rôle ambivalent des symétries dans les problèmes d’inférence Bayesienne]
Nous regroupons dans cette note quelques observations sur le rôle des symétries dans les problèmes d’inférence Bayesienne, qui peuvent être utiles ou néfastes selon la façon dont elles agissent sur le signal et les observations. Nous soulignons en particulier la nécessité de quotienter les invariances non-observables dans la définition d’une distance entre un signal et son estimateur, et les conséquences que ceci implique pour le traitement par la mécanique statistique de tels modèles, motivé par l’exemple de la factorisation de matrices de rang extensif.
We collect in this note some observations on the role of symmetries in Bayesian inference problems, that can be useful or detrimental depending on the way they act on the signal and on the observations. We emphasize in particular the need to gauge away unobservable invariances in the definition of a distance between a signal and its estimator, and the consequences this implies for the statistical mechanics treatment of such models, taking as a motivating example the extensive rank matrix factorization problem.
Révisé le :
Accepté le :
Publié le :
Mots-clés : Problèmes d’inférence Bayesienne, Symétries, Mécanique statistique des systèmes désordonnés
Guilhem Semerjian 1
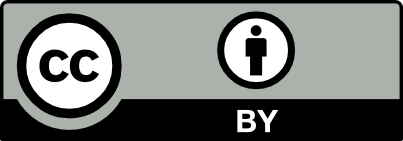
@article{CRPHYS_2025__26_G1_199_0, author = {Guilhem Semerjian}, title = {Some observations on the ambivalent role of symmetries in {Bayesian} inference problems}, journal = {Comptes Rendus. Physique}, pages = {199--216}, publisher = {Acad\'emie des sciences, Paris}, volume = {26}, year = {2025}, doi = {10.5802/crphys.236}, language = {en}, }
Guilhem Semerjian. Some observations on the ambivalent role of symmetries in Bayesian inference problems. Comptes Rendus. Physique, Volume 26 (2025), pp. 199-216. doi : 10.5802/crphys.236. https://comptes-rendus.academie-sciences.fr/physique/articles/10.5802/crphys.236/
[1] Representation Theory, a First Course, Springer, New York, 1991 | DOI
[2] Symmetry, Representations, and Invariants, Springer, New York, 2009 | DOI
[3] Group Theory in a Nutshell for Physicists, Princeton University Press, Princeton, 2016
[4] Theory of frustration effect in spin-glasses: I, Commun. Phys., Volume 2 (1977), pp. 115-119 | DOI
[5] Symmetry and topology concepts for spin glasses and other glasses, Phys. Rep., Volume 49 (1979), pp. 267-272 | DOI
[6] Group invariance applications in statistics, Regional Conference Series in Probability and Statistics, 1, Institute of Mathematical Statistics, Hayward, 1989, pp. i-133 | DOI
[7] Invariant measures on groups and their use in statistics, Lecture Notes-Monograph Series, 14, Institute of Mathematical Statistics, Hayward, 1990, pp. i-218
[8] Theory of Point Estimation, Springer, New York, 1998 | DOI
[9] Building symmetries into feedforward networks, First IEE International Conference on Artificial Neural Networks, (Conf. Publ. No. 313), IET, London, 1989, pp. 158-162
[10] Geometric deep learning: grids, groups, graphs, geodesics, and gauges, preprint, 2021 | arXiv
[11] Towards fully covariant machine learning, Trans. Mach. Learn. Res. (2024) (submitted)
[12] Group equivariant convolutional networks, Proceedings of The 33rd International Conference on Machine Learning (M. F. Balcan; K. Q. Weinberger, eds.) (Proceedings of Machine Learning Research, PMLR), Volume 48, PMLR, New York, 2016, pp. 2990-2999
[13] A group-theoretic framework for data augmentation, J. Mach. Learn. Res., Volume 21 (2020) no. 245, pp. 1-71 | Zbl
[14] Geometry of the loss landscape in overparameterized neural networks: symmetries and invariances, Proceedings of the 38th International Conference on Machine Learning (M. Meila; T. Zhang, eds.) (Proceedings of Machine Learning Research, PMLR), Volume 139, PMLR, New York, 2021, pp. 9722-9732
[15] Statistical Physics of Spin Glasses and Information Processing: An Introduction, Oxford University Press, Oxford, 2001 | DOI
[16] Statistical Mechanics of Learning, Cambridge University Press, Cambridge, 2001 | DOI
[17] Physics, Information, Computation, Oxford University Press, Oxford, 2009 | DOI
[18] Statistical physics of inference: thresholds and algorithms, Adv. Phys., Volume 65 (2016) no. 5, pp. 453-552 | DOI
[19] Spin Glass Theory and Far Beyond (P. Charbonneau; E. Marinari; M. Mézard; G. Parisi; F. Ricci-Tersenghi; G. Sicuro; F. Zamponi, eds.), World Scientific, Singapore, 2023 | DOI
[20] Categorical data analysis of single sociometric relations, Sociol. Methodol., Volume 12 (1981), pp. 156-192 | DOI
[21] Stochastic blockmodels: first steps, Social Networks, Volume 5 (1983) no. 2, pp. 109-137 | DOI
[22] The phase transition in inhomogeneous random graphs, Random Struct. Algorithms, Volume 31 (2007) no. 1, pp. 3-122 | DOI | Zbl
[23] Asymptotic analysis of the stochastic block model for modular networks and its algorithmic applications, Phys. Rev. E, Volume 84 (2011), 066106 | DOI
[24] The computer science and physics of community detection: landscapes, phase transitions, and hardness, Bull. EATCS, Volume 1 (2017) no. 121 | Zbl
[25] Community detection and stochastic block models: recent developments, J. Mach. Learn. Res., Volume 18 (2018) no. 177, pp. 1-86 | Zbl
[26] Rotational invariant estimator for general noisy matrices, IEEE Trans. Inf. Theory, Volume 62 (2016) no. 12, pp. 7475-7490 | DOI | Zbl
[27] Perturbative construction of mean-field equations in extensive-rank matrix factorization and denoising, J. Stat. Mech.: Theory Exp., Volume 2022 (2022) no. 8, 083301 | DOI | Zbl
[28] Statistical limits of dictionary learning: Random matrix theory and the spectral replica method, Phys. Rev. E, Volume 106 (2022), 024136 | DOI
[29] Optimal denoising of rotationally invariant rectangular matrices, Proc. Math. Sci. Mach. Learn. (MSML), Volume 190 (2022), pp. 97-112
[30] Matrix inference in growing rank regimes, IEEE Trans. Inform. Theory, Volume 70 (2024) no. 11, pp. 8133-8163 | DOI | Zbl
[31] Matrix denoising: bayes-optimal estimators via low-degree polynomials, J. Stat. Phys., Volume 191 (2024), 139 | DOI | Zbl
[32] On the phase diagram of extensive-rank symmetric matrix denoising beyond rotational invariance, preprint, 2024 | arXiv
[33] Emergence of simple-cell receptive field properties by learning a sparse code for natural images, Nature, Volume 381 (1996), pp. 607-609 | DOI
[34] Blind source separation by sparse decomposition in a signal dictionary, Neural Comput., Volume 13 (2001) no. 4, pp. 863-882 | DOI | Zbl
[35] Dictionary learning algorithms for sparse representation, Neural Comput., Volume 15 (2003) no. 2, pp. 349-396 | DOI | Zbl
[36] Exact recovery of sparsely-used dictionaries, Proceedings of the 25th Annual Conference on Learning Theory, Volume 23, PMLR, New York, 2012, p. 37.1-37.18
[37] Iterative estimation of constrained rank-one matrices in noise, 2012 IEEE International Symposium on Information Theory Proceedings, IEEE, Cambridge, 2012, pp. 1246-1250 | DOI
[38] Mutual information for symmetric rank-one matrix estimation: A proof of the replica formula, Advances in Neural Information Processing Systems (D. Lee et al., eds.), Volume 29, Curran Associates, Inc., Red Hook, 2016, pp. 424-432 https://proceedings.neurips.cc/paper_files/paper/2016/file/621bf66ddb7c962aa0d22ac97d69b793-Paper.pdf (accessed February 10, 2025)
[39] Constrained low-rank matrix estimation: phase transitions, approximate message passing and applications, J. Stat. Mech.: Theory Exp., Volume 2017 (2017) no. 7, 073403 | DOI | Zbl
[40] Fundamental limits of symmetric low-rank matrix estimation, Probab. Theory Relat. Fields, Volume 173 (2019), pp. 859-929 | DOI | Zbl
[41] The adaptive interpolation method: a simple scheme to prove replica formulas in Bayesian inference, Probab. Theory Relat. Fields, Volume 174 (2019), pp. 1133-1185 | DOI | Zbl
[42] Equivalence of approximate message passing and low-degree polynomials in rank-one matrix estimation, preprint, 2022 | arXiv
[43] Statistical mechanics of dictionary learning, Europhys. Lett., Volume 103 (2013) no. 2, 28008 | DOI
[44] Phase transitions and sample complexity in bayes-optimal matrix factorization, IEEE Trans. Inf. Theory, Volume 62 (2016) no. 7, pp. 4228-4265 | DOI | Zbl
[45] Statistical physics of sparse and dense models in optimization and inference, PhD Thesis, Université Paris-Saclay (2018)
[46] A multiscale cavity method for sublinear-rank symmetric matrix factorization, preprint, 2024 | arXiv
[47] Bayesian extensive-rank matrix factorization with rotational invariant priors, Advances in Neural Information Processing Systems (A. Oh et al., eds.), Curran Associates Inc., Red Hook, 2023, pp. 24025-24073 https://proceedings.neurips.cc/paper_files/paper/2023/file/4b8afc47273c746662a96dfdf562f87f-Paper-Conference.pdf (accessed February 10, 2025)
[48] Rectangular rotational invariant estimator for general additive noise matrices, 2023 IEEE International Symposium on Information Theory (ISIT), Taipei, Taiwan, IEEE, Cambridge, 2023, pp. 2081-2086 | DOI
[49] Singular vectors of sums of rectangular random matrices and optimal estimation of high-rank signals: The extensive spike model, Phys. Rev. E, Volume 108 (2023), 054129 | DOI
[50] Matrix factorization with neural networks, Phys. Rev. E, Volume 107 (2023), 064308 | DOI
[51] The decimation scheme for symmetric matrix factorization, J. Phys. A: Math. Theor., Volume 57 (2024) no. 8, 085002 | DOI | Zbl
[52] The Elements of Statistical Learning, Springer, New York, 2009 | DOI
[53] Multivariate priors and the linearity of optimal Bayesian estimators under Gaussian noise, 2024 IEEE International Symposium on Information Theory (ISIT), Athens, Greece, IEEE, Cambridge, 2024, pp. 987-992 | DOI
[54] Symmetric Functions and Hall Polynomials, Oxford University Press, Oxford, 1998
[55] The Classical Groups: Their Invariants and Representations, Princeton University Press, Princeton, 1966
[56] Computational barriers to estimation from low-degree polynomials, Ann. Stat., Volume 50 (2022) no. 3, pp. 1833-1858 | DOI | Zbl
[57] Efficient bayesian estimation from few samples: community detection and related problems, 2017 IEEE 58th Annual Symposium on Foundations of Computer Science (FOCS), IEEE, Cambridge, 2017, pp. 379-390 | DOI
[58] Notes on computational hardness of hypothesis testing: predictions using the low-degree likelihood ratio, Mathematical Analysis, its Applications and Computation, Springer International Publishing, Cham, 2022, pp. 1-50 | Zbl
[59] Tensor cumulants for statistical inference on invariant distributions, 2024 IEEE 65th Annual Symposium on Foundations of Computer Science (FOCS), Chicago, IL, USA, IEEE, Cambridge, 2024, pp. 1007-1026 | DOI
[60] Learning with invariances in random features and kernel models, Proceedings of Thirty Fourth Conference on Learning Theory (M. Belkin; S. Kpotufe, eds.) (Proceedings of Machine Learning Research, PMLR), Volume 134, PMLR, New York, 2021, pp. 3351-3418
[61] Estimation in the group action channel, 2018 IEEE International Symposium on Information Theory (ISIT), IEEE, Cambridge, 2018, pp. 561-565 | DOI
[62] Estimation under group actions: recovering orbits from invariants, Appl. Comput. Harmon. Anal., Volume 66 (2023), pp. 236-319 | DOI | Zbl
[63] Complexity of robust orbit problems for torus actions and the abc-conjecture, 39th Computational Complexity Conference (CCC 2024) (R. Santhanam, ed.) (Leibniz International Proceedings in Informatics (LIPIcs)), Volume 300, Schloss Dagstuhl – Leibniz-Zentrum für Informatik, Dagstuhl, Germany, 2024, p. 14:1-14:48 | DOI
[64] Cryo-electron microscopy of viruses, Nature, Volume 308 (1984), pp. 32-36 | DOI
[65] Three-dimensional structure determination from common lines in Cryo-EM by eigenvectors and semidefinite programming, SIAM J. Imaging Sci., Volume 4 (2011) no. 2, pp. 543-572 | DOI | Zbl
[66] A maximum-likelihood approach to single-particle image refinement, J. Struct. Biol., Volume 122 (1998) no. 3, pp. 328-339 | DOI
[67] Inequalities, Cambridge Mathematical Library, Cambridge University Press, Cambridge, 1934
[68] Theoretical improvements in algorithmic efficiency for network flow problems, J. ACM, Volume 19 (1972) no. 2, pp. 248-264 | DOI | Zbl
[69] Mutual information and minimum mean-square error in Gaussian channels, IEEE Trans. Inf. Theory, Volume 51 (2005) no. 4, pp. 1261-1282 | DOI | Zbl
[70] Stochastic optimization by message passing, J. Stat. Mech.: Theory Exp., Volume 2011 (2011) no. 11, P11009 | DOI
[71] Adversarial satisfiability problem, J. Stat. Mech.: Theory Exp., Volume 2011 (2011) no. 03, P03023 | DOI
[72] Max-product for maximum weight matching: convergence, correctness, and LP duality, IEEE Trans. Inform. Theory, Volume 54 (2008) no. 3, pp. 1241-1251 | DOI | Zbl
[73] A mean field view of the landscape of two-layer neural networks, Proc. Natl. Acad. Sci., Volume 115 (2018) no. 33, p. E7665-E7671 | Zbl
[74] Marvels and pitfalls of the langevin algorithm in noisy high-dimensional inference, Phys. Rev. X, Volume 10 (2020), 011057 | DOI
[75] Online stochastic gradient descent on non-convex losses from high-dimensional inference, J. Mach. Learn. Res., Volume 22 (2021) no. 106, pp. 1-51 | Zbl
[76] Noether: the more things change, the more stay the same, preprint, 2021 | arXiv
[77] On the symmetries in the dynamics of wide two-layer neural networks, Electron. Res. Arch., Volume 31 (2023) no. 4, pp. 2175-2212 | DOI
[78] The benefits of reusing batches for gradient descent in two-layer networks: breaking the curse of information and leap exponents, Proceedings of the 41st International Conference on Machine Learning (ICML’24), JMLR.org, Vienna, 2025
Cité par Sources :
Commentaires - Politique