1 Introduction
The models used for resources assessment rarely take into account the total life cycle of an exploited marine population. Often, they only consider the individuals susceptible to exploitation, which constitute the so-called stock. The exploited stock does not contain in general larvas and old fish, because larvas and alevins are too small or absent in the potential fishing zones, and the old fish eventually leave the fishing zones, or become inaccessible to the fleet. But we notice that the fishers do not exclude the fishing of the juvenile, and that it is developing in an alarming way and without control even if there are strict measures that forbid this fishing, therefore to solve this problem, there must take in consideration this fishing with taking in account the juvenile stage in the system that describes the stock evolution.
In building a model of a resource it is necessary to define variables which adequately describe the state of the resource at any time. Such variables are called state variables. For renewable resources they often describe a ‘standing stock’, frequently the number of individuals in a population or the ‘biomass’ of the population. If the age structure, sex ratio, or other population characteristics are important, the model will require more than one state variable. In the literature, models representing the evolution of a stock exploited, are divided in two groups: global models [1–8] and structured models [9–13]; the first one presents the stock as a unique variable, whereas, the second distinguishes between several stages (classes of ages, of size...) of the stock and associates with each one of them a dynamical variable.
So, the global models give a general vision of the stock evolution. But, the responsible authorities of the fishing management may be interested in the impact of certain technical measures like for instance, the reduction of the mesh's fishing nets. The structured models are able to respond to this type of investigations. They permit a qualitative description of the system since they take into account both features: the fish size and the time mechanism of reproduction of the exploited stock.
The objective of this work is to find an optimal strategy of the fishing problem based on a structured model. The case of a dynamics following from a global model is a classic one, and the research of an optimal exploitation policy has been the object of several articles (see, for instance, Clark [1], Clark et al. [2], Jerry and Raïsi [14,15], Raïssi [16]). Section 2 is devoted to the formulation of the fishing problem. In Section 3, we apply the maximum principle to the resulting fishing problem. In Section 4 we present the optimal strategy exploitation.
2 Presentation of the fishing model
Our objective consists on the study of a structured model containing a stage of juvenile. In some previous studies, the recruiting stage is formulated either as a constant or as noise. The restrictive feature of this approach is that the stock – recruitment relationship does not appear. On the other hand, there exist stock – recruitment relationships in literature, for example, the model of Deriso [17], generalized by Schnute [18], Model depositories [19], etc. In this work we are inspired by the models used by Ricker [11,12], Beverton and Holt [9,10], and Touzeau [13] because they are synthetic and mathematically tractable. Even without data on the previous stages of the recruitment, these equations remain a useful tool for the assessment of the stocks [20].
More precisely, our dynamic model is a continuous time model with two states: the juvenile and the adult, where every stage is described by the evolution of its biomass and , respectively.
(2.1) |
or corresponds to the extinction of the species, because if we have , according to the first equation of the system (2.1), we will have , but is a non-null constant, then , in the other case, if we have , according to the second equation of the system (2.1), we will have , but α is a non-null constant, then .Remark 2.1
Each stage i of the stock suffers a mortality rate, due to fishing and natural disaster. The natural mortality incorporates diseases, perturbations generated by the environment and by other species outside the stock, in other words, all factors except the human exploitation and the interactions within the stock. We assume that this mortality is linear (constant rate ). The ageing is also supposed to be linear. On the other hand, the passage rate α from the juvenile class to the adult stage is supposed to be constant with respect to time and stage. This means that the time of residence is equal to .
We assume that the laying (eggs) is continuous with respect to time, this assumption constituting a simplification in the model. The species egg laying periods may take place several times per year, even continuously. The number of viable eggs (in the unit of time) introduced in the juvenile stage is given by , where is the mean number of eggs deposited by fertile adult in the unit of time, and is the number of adults.
Let us, first note that, according to their definition (mortality rate, fishing effort...), all parameters in the model are nonnegative. For a correct representation of a structured population in the model, we must take into account the recruitment from one class to another, which can be represented by a strictly positive coefficient of passage.
Now assume that the price, , of the harvested resource is a fixed constant; furthermore assume that the cost, , of a unit of fishing effort is also constant. Then the sole owner's objective is the maximization of the total discounted net revenues derived from exploitation of the resource. We suppose that there exists only one decision-maker of the fishing, who can fish both the juvenile and the adults. If is a constant denoting the (continuous) rate of discount, this objective may be expressed as maximizing
(2.2) |
(2.3) |
In this model, the fishing of the juvenile and larvas are not excluded, since we have suppose that the constants , , and are positive. We suppose that:
(2.4) |
It is true that we maximize also the net revenues derived by the exploitation of juvenile (2.2) but the constraints imposed by the problem (2.4) do not encourage this fishing so this revenues is not very significant compared to the revenues generated by the exploitation of adults. With the above formulation, the fishing problem is viewed as an optimal control problem. Our goal consists on the determination of an optimal fishing effort, , subject to (2.3) and (2.4) such that if is the corresponding solution of the state system, then maximizes the total discounted net revenues generated by the exploitation of the stock over all admissible processes satisfying (2.1), (2.3) and (2.4).
3 Application of the maximum principle
The data of the model introduced in the previous section satisfies the required standing hypotheses for the application of the maximum principle [21,22]. First we introduce the Hamiltonian:
(3.1) |
(3.2) |
If we replace R by , and S by , the associate system (3.2) becomes:
(3.3) |
The Hamiltonian become
(3.4) |
The Pontryagin's maximum principle provides a necessary optimality condition of . For all t, must maximize the Hamiltonian. The linearity of the Hamiltonian with respect to the controls leads to a ‘bang–bang’ optimal control: a control that takes on these extreme values is called a bang–bang control
(3.5) |
However, note that when the switching function or vanishes, the Hamiltonian becomes independent of , so the maximum principle does not specify the value of the optimal control. The most important case (called the singular case) arises when or vanishes identically over some time interval of positive length. Establishing the existence of this interval will permit us to identify the following system by deriving these two equations and :
(3.6) |
The system (3.6) can admit some solutions, with respect to the parameter values of the problem. Our study will take place in the case where the system (3.6) does not admit any solutions, but with parameter values coming from the literature [13].
The object of the next section is to describe and to prove the optimal strategy of the problem.
4 The optimal strategy
By using the results of the previous section, we are ready to describe definitively the optimal exploitation policy. Now we study the system (3.6) given in the previous section. Let us the following function denote by , the first equation of system (3.6):
(4.1) |
Eq. (4.1) admits two real roots, the first one is positive, and the second is negative, we are interested by the first one:
(4.2) |
The graph of the function is given by Fig. 1.
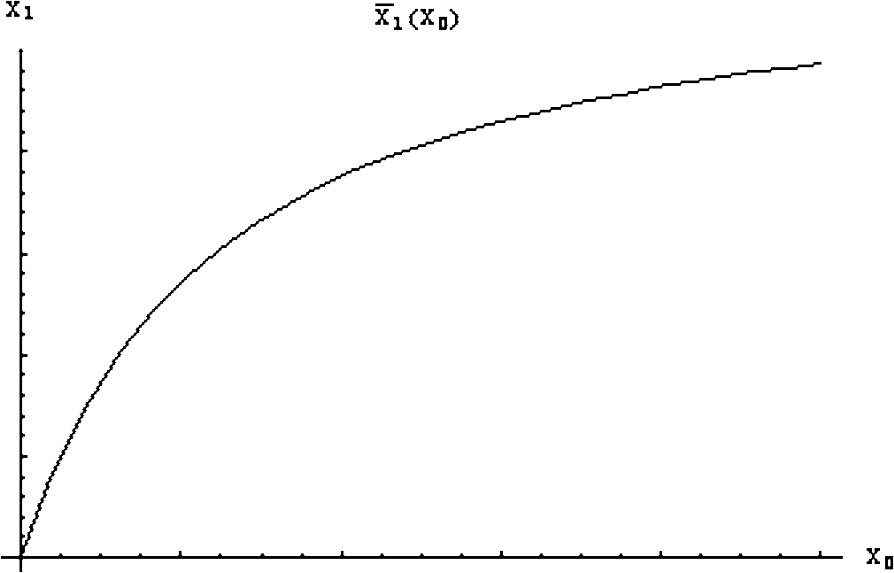
The graph of the function for the following values: α=0.8, , , , , , δ=0.2, , , , .
Consider now the second equation of system (3.6):
(4.3) |
Eq. (4.3) admits two real roots, the first one is positive, and the second is negative, likewise we are interested by the first root:
(4.4) |
The graph of the function is given by Fig. 2.
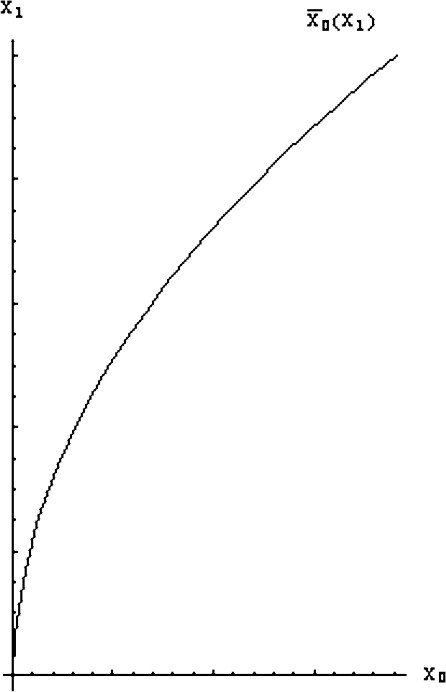
The graph of the function for the following values: α=0.8, , , , , , δ=0.2, , , , .
These parameters are from [13], even if they not correspond at any real stock. Their value depends of the units reserved for the variables of the model, for example, depends of the unit of time (year, month...), of the number of the stock (in thousand, millions...).
The preceding analysis of the two equations will permit us to prove the two following lemmas:
Consider
, if
, then the optimal strategy is
. Otherwise, if
, we have
.
Lemma 4.1
Consider
, if
, then the optimal strategy is
. Otherwise, if
, we have
.
Lemma 4.2
Taking into account the previous lemmas, we can now describe the optimal strategy that we must follow for any . This optimal strategy is given and illustrate by the following diagram and Fig. 3, respectively:
(4.5) |

Determination of optimal strategy.
We remark that the two curves and do not intersect. if we are above the curve , the juvenile biomass is weak and the adult biomass is large. The optimal control consists of taking in order to increase the juvenile biomass as fast as possible until the trajectory reaches the area delimited by the two curves and to take as optimal control . On the other hand, if we are under the curve , the adult biomass is weak and the juvenile biomass is large. The optimal control consists of taking in order to increase the adult biomass as fast as possible until the trajectory reaches the area delimited by the two curves and to take as optimal control .
It is easy to show that the optimal strategy described above is a bang–bang strategy, and it is very simple to apply because, according to the position of towards two curves , on the other hand if we are on one of the two curves, the corresponding optimal strategy is any value of according to the principle of the maximum especially Eq. (3.4). We deduct the feasible optimal policy to our fishing problem.
5 Conclusion
In the previous results [1,9,10,13], the aim was the search of equilibrium points for a structured model and the study of the stability (saddle point, stable and unstable node, stable and unstable focus, center...), but in this work, a structured model is associated with the maximization of a total discounted net revenues derived from exploitation of the resource, and the main objective is to prove the existence of an optimal strategy for the fishing problem. By using the tools of the control theory, in particular, the Pontryagin's maximum principle, we are found the optimal strategy of the fishing problem.
One drawback of the model is that it does not take into consideration any intraspecific competition between species, the competition between the juvenile and the predation of the adults on the small; It is also a structured model only of two states, therefore it would be necessary to see what happens for a model of dimension , besides we work with constant parameters during the time, but it does not prevent that all results of this work are interesting since they constitute a basis of reflection and they are a valuable data for new works.